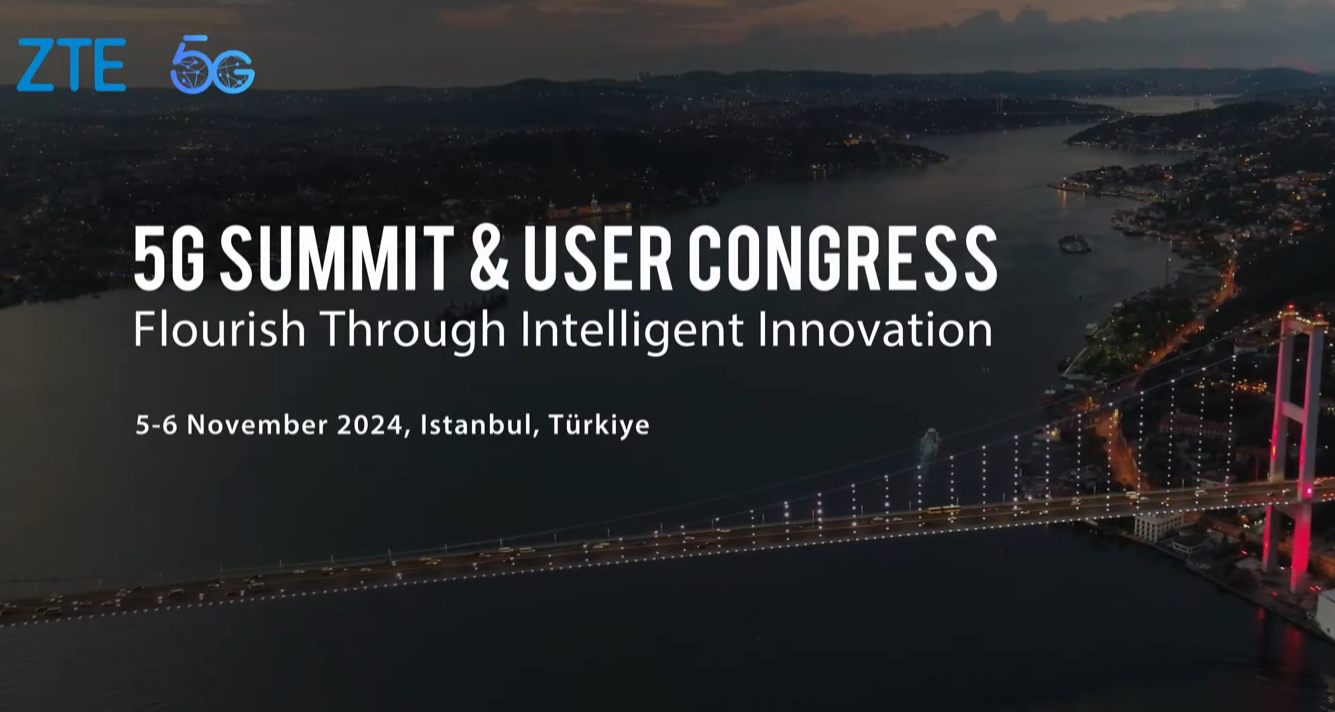
Introduction
Introduction During the 5G Summit & User Congress 2024 held in Istanbul, Turkey, ZTE Corporp. introduced its innovative AI OpsBot Core Network Intelligent Expert. This advanced solution is set to transform network operations and maintenance (O&M) by leveraging a combination of large and small AI models alongside intelligent agent coordination. The result is a significant improvement in efficiency compared to conventional O&M approaches.
With telecommunications networks growing increasingly intricate, AI-powered tools like the AI OpsBot are becoming essential. They enable greater automation and enhanced network management capabilities. The unveiling of this technology aligns with the summit’s focus, “Flourish Through Intelligent Innovation,” highlighting AI’s pivotal role in shaping the future of 5G and beyond.
ZTE Corp. AI OpsBot: Transforming Core Network Operations
ZTE Corp.’s AI OpsBot Core Network Intelligent Expert is engineered to revolutionize core network operations by leveraging advanced AI to streamline and optimize O&M tasks. Acting as the central hub for network management, the AI OpsBot combines large and small AI models to process intricate datasets and provide real-time decision-making capabilities. This dual-model approach enables it to address a broad spectrum of network challenges, from high-level performance monitoring to detailed troubleshooting.
A standout feature is the system’s intelligent agent orchestration, which ensures seamless collaboration across multiple network layers. These agents dynamically adapt to evolving conditions, enabling continuous optimization without human intervention. By automating tasks such as fault detection, performance analysis, and diagnostics, the AI OpsBot reduces troubleshooting time, enhances accuracy, and minimizes errors. This leads to more efficient network operations, faster issue resolution, and reduced downtime, fostering a highly reliable core network.
Role of Large and Small AI Models in Network Operations
The AI OpsBot’s strength lies in its integration of large and small AI models, each tailored to specific functions, creating a comprehensive solution for network management.
How Large AI Models Function
Large AI models excel in intelligent human-machine interaction and broad automation. Their adaptability allows them to operate across diverse network environments without requiring task-specific customization. These models handle tasks such as generating detailed performance reports and analyzing large-scale network data, enabling operators to make informed decisions efficiently. Additionally, they support natural language processing (NLP), allowing intuitive, conversational interactions with the system, simplifying operator workflows.
How Small AI Models Operate
Small AI models are designed for precision and specificity, excelling in tasks like anomaly detection, key performance indicator (KPI) forecasting, and mitigating issues such as signaling storms. By leveraging historical data, these models predict potential performance issues, such as bandwidth constraints or latency spikes, enabling proactive measures. Their ability to pinpoint faults with high accuracy significantly reduces troubleshooting time and enhances network reliability.
The Impact of Automation
The combination of large and small AI models enables the AI OpsBot to automate critical tasks like fault identification, performance monitoring, and KPI prediction. This synergy enhances network reliability, reduces manual oversight, and accelerates issue resolution, resulting in a more responsive and intelligent network management system.
Achieving 30% Efficiency Gains with Minimal Human Intervention
Traditional O&M processes in telecom networks rely heavily on manual efforts to monitor performance, diagnose issues, and implement solutions. These methods are often time-consuming, error-prone, and difficult to scale in complex, data-intensive environments. The AI OpsBot addresses these challenges by automating tasks across network layers through its intelligent agent framework, powered by large and small AI models.
ZTE reports that the AI OpsBot delivers a 30% improvement in operational efficiency compared to conventional O&M methods. This is achieved by enabling intelligent agents to autonomously manage complex tasks, reducing the need for human involvement. The system’s automation capabilities improve accuracy, speed up issue resolution, and lower operational costs by minimizing resource demands. This allows telecom operators to focus on strategic initiatives rather than routine maintenance.
The adoption of AI-driven solutions like the AI OpsBot has significant implications for the telecom industry. Enhanced network reliability reduces service disruptions, improving customer satisfaction. Additionally, lower staffing requirements for O&M tasks translate to cost savings. As ZTE advances toward L4-level intelligent O&M, the AI OpsBot sets a new standard for efficiency and stability in telecommunications.
Conclusion
ZTE’s AI OpsBot Core Network Intelligent Expert represents a major milestone in network O&M automation. By integrating large and small AI models, the system delivers unmatched efficiency, reduced costs, and enhanced network performance. The reported 30% efficiency improvement highlights AI’s transformative potential in the telecom sector. As AI technology continues to evolve, it will play an increasingly vital role in minimizing errors, optimizing operations, and enabling telecom providers to meet the demands of a rapidly growing digital ecosystem.
Main Reference: zte.com.cn/global/about/news/zte-showcases-ai-opsbot-core-network-intelligent-expert-at-5g-summit-user-congress-2024.html